New Insights from Old Numbers: Artificial Intelligence Makes Sense of Health Ministry Data
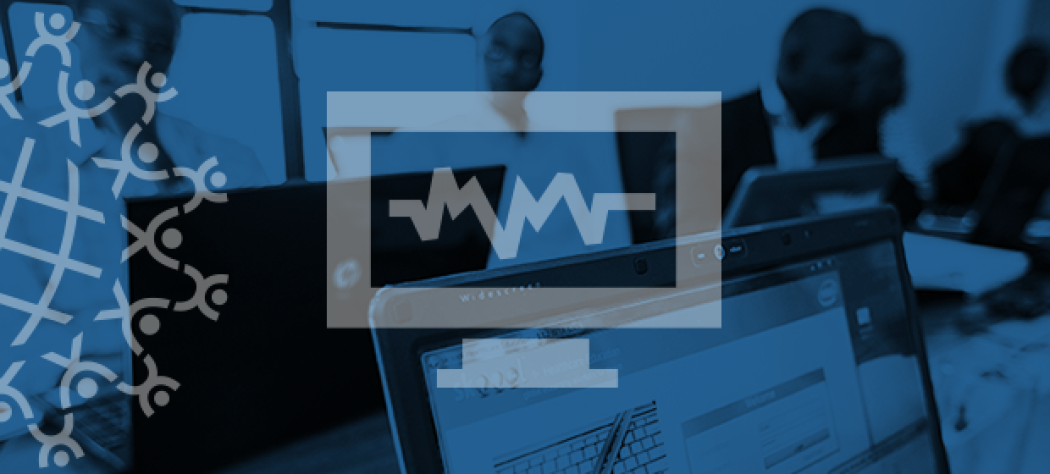
Ministries of health have more data than ever before—and the amount of data is increasing at an exponential rate. But what good is all that information if it’s unwieldy or difficult to use?
Over the course of nine weeks, IntraHealth International’s digital health team and staff from our Regional Health Integration to Enhance Services in Eastern Uganda (RHITES-E) project modeled a machine-learning approach to glean insights from the flood of health systems and population data available to the Ugandan ministry of health. What they found will help ministry officials make more strategic decisions about the support they allocate to health facilities—making questions around which facilities need what and when more clear-cut.
“We collect vast amounts of data,” says Nicholas Matsiko, RHITES-E monitoring and evaluation manager. “This is a big step in solving the data-rich-but-information-poor paradigm facing ministries of health.”
Machine learning is an application of artificial intelligence that enables data systems to learn from experience without being directly programmed. When it’s applied in this way, machine learning helps clarify and summarize data, removing unhelpful variables from the data pool and highlighting those that are most informative.
After pulling data from several sources, including program data from the RHITES-E project, the census, the Demographic and Health Survey, DHIS2, and iHRIS, IntraHealth’s team used what’s called “unsupervised” machine learning. All variables—in this case, 1,200—were put into a model for grouping. This process reveals hidden patterns and brings to light the underlying phenomena under the indicators. Why, for example, do Health Facility A and Health Facility B have enough in common to be clustered together in the model even though they have markedly different levels of HIV treatment retention?
IntraHealth used a second “supervised” machine learning step to narrow the scope of variables that are necessary to describe the differences between facilities, reducing them from 1,200 to just 38. Clearing away unhelpful variables helps more actionable ones, like the number of live births at a facility or the availability of equipment and supplies, stand out to ministry officials.
The team is keen to continue the process in Uganda and apply it in other countries.
“We now have a framework that we can use to support ministries to become more data-driven,” says IntraHealth senior data scientist Amy Finnegan, “and make sense of the data they already have.”