What Happens When Global Health and Data Science Collide?
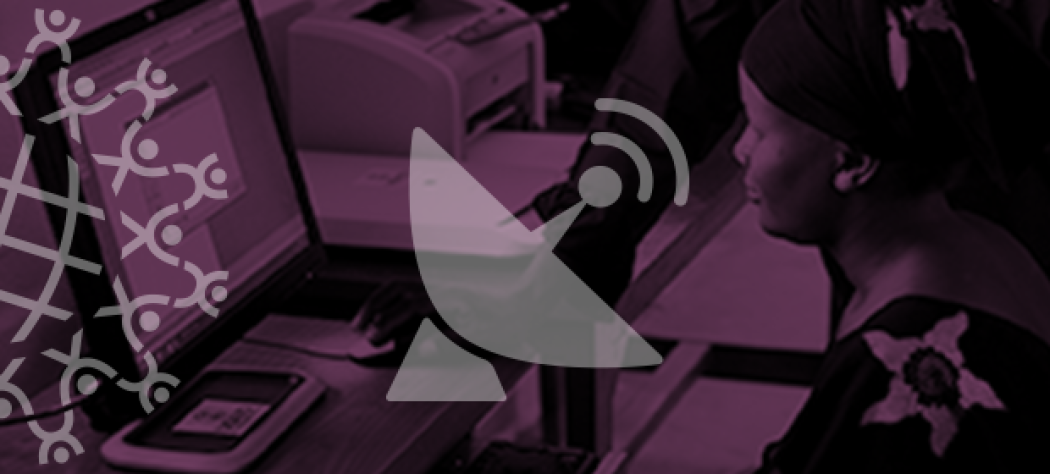
Data scientist Amy Finnegan’s job is to ask the right questions to get new answers. She uses machine learning to solve global health puzzles like “Why do women who use contraception in low-income countries stop using if they still want to avoid pregnancy?” and “What elements of populations and geography can determine whether a health program is successful?”
We sat down with her to ask how this work is shaping the future of global health—and what comes next.
What's your favorite thing about working in this field?
I have always been fascinated with human experiences like births, deaths, and migration and the behaviors that drive choices and help or inhibit people from converting their intentions into action.
So often those behaviors and choices have implications for global health. I think of global health not as a discipline, but as a collection of problems that require many different approaches.
Machine learning helps us decide what’s important to the human experience.
It’s an old approach from computer science that has been unlocked for global health because there is so much data available.For example, we’ve been using machine learning in our team’s pilot work in Uganda to quickly find new insights—finding different groupings we didn’t know existed in old data. Machine learning algorithms are really teaching us to challenge traditional approaches to improve and save human lives.
What's one thing you think people should know about data science in global health?
The real value of data isn’t how much you have but how you use them.